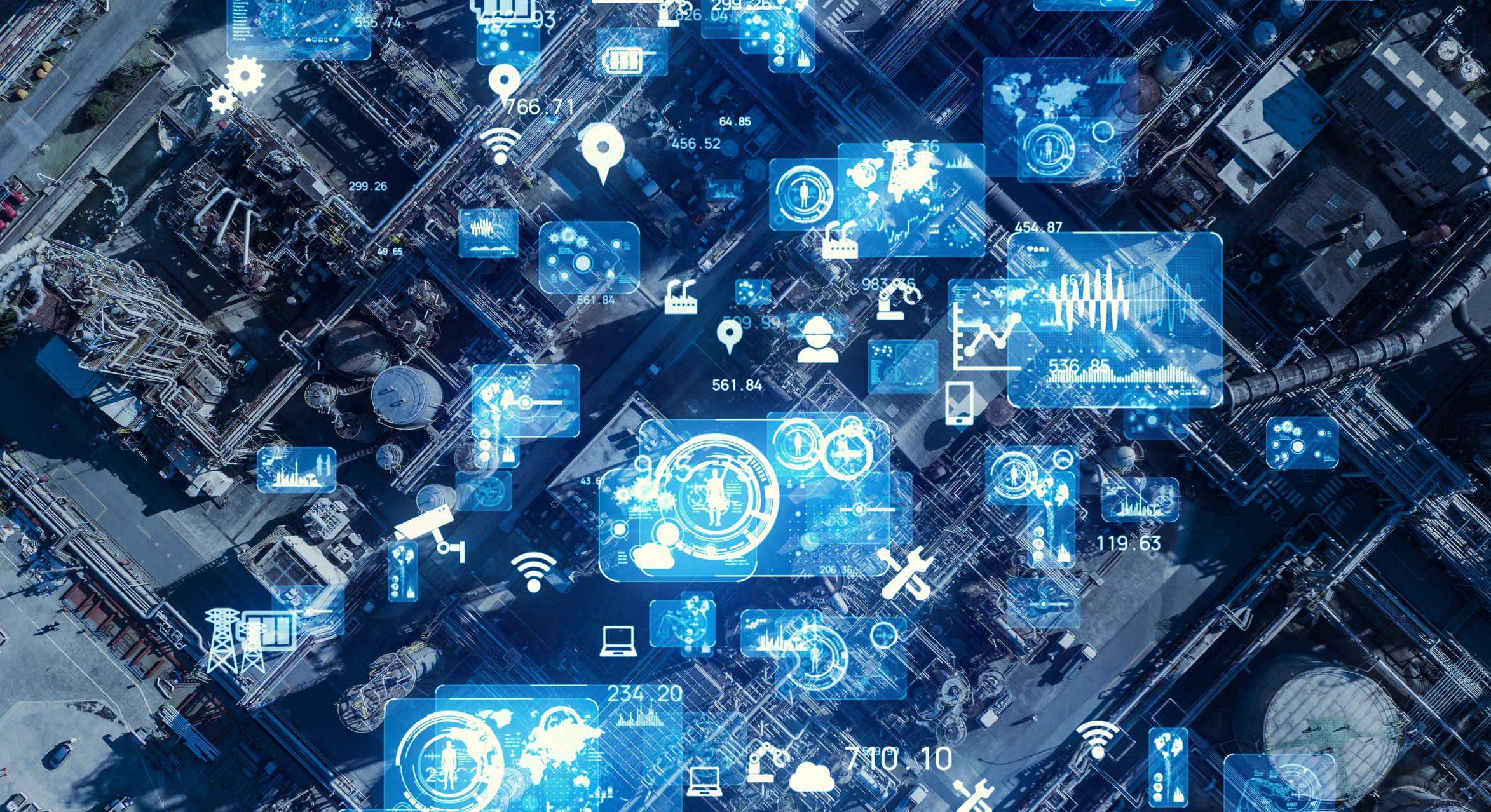
Of all the tech trends on the horizon, Artificial Intelligence is the one that has most thoroughly captured the collective imagination. And hey, we get it. Modern computers are capable of truly stunning feats, whether it’s the immersive virtual environments of Building Information Modeling, or the seamless integration of our very own ONE-KEY™ tool tracking and inventory management platform.
Our team of electrical and computer engineers here at Milwaukee® Tool are no strangers to AI research; in collaboration, they’ve successfully deployed machine-learning to improve tracking and prevent kickback in our line of One-Key compatible smart tools. As excited as we are about the role AI in construction already plays, we also recognize that it is the source of a lot of understandable confusion and anxiety. That’s why we’ve done our best to explain what modern AI both is and is not, while also being specific and transparent about how exactly we are using it.
But no honest or grounded account of AI would be complete without a reckoning with some of its pitfalls. In this article, we will lay out some common criticisms of AI and attempt to illuminate a path into both a present and future world where this transformative technology may be used safely to the benefit of everyone, whether on the jobsite or off it.
AI Ethics Examples: What Are the 5 Major AI Issues?
What are the ethical issues of AI?
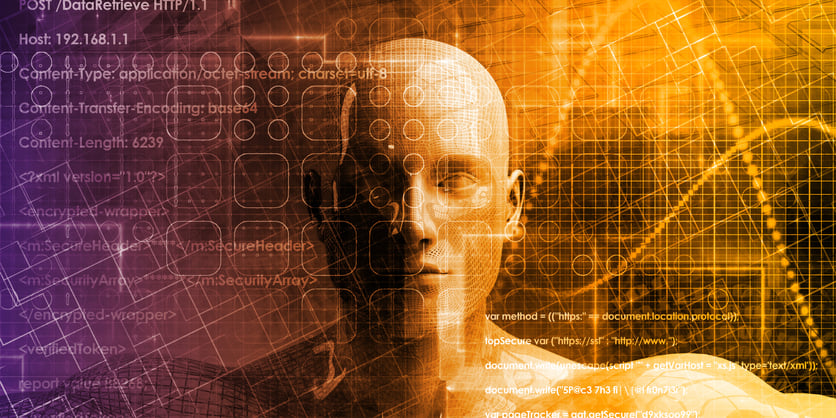
Here are 5 AI ethics examples of problems that need to be addressed with artificial intelligence technology:
- Ambiguous Terminology
- Over-hyped Technology
- Artificial Bias
- The Black Box Problem
- Uncertainty
Ambiguous Terminology
A major problem with artificial intelligence is that it means so many different things to so many different people. Given the maelstrom of buzzwords, opinions, media depictions, and debates surrounding AI, it can be difficult to know what the conversation is really about at any given time. Are we talking about machine-learning or deep-learning? Weak AI, otherwise known as Artificial Narrow Intelligence (ANI) or Strong AI, also referred to as Artificial General Intelligence (AG)? Reactive Machines or Limited Memory machines? Theory of Mind AI or Self-Aware AI? Symbolic AI or Superintelligent AI?
We’ve attempted to simplify and explain a handful of these terms in a previous article. But as you can see, it’s easy to get confused with so many ideas about what AI is (or should be) flying through the ether. Alternate yet related definitions pass each other like ships in the night, and it sometimes seems like anything that has anything to do with computers is an example of AI these days. To muddy the waters even further, AI terms are wide open to interpretation, and often get conflated with one another, blurring the line between science-fiction and science-reality. Whether by accident or on purpose, promoters of AI sometimes end up taking advantage of this ambiguity, promising superintelligent performance from modern machine-learning systems that are currently only adept at executing narrow sets of tasks.
Which brings us to our next critique of artificial intelligence: the hype.
Hype Machines
Artificial Intelligence is perhaps the most overhyped technology on the planet right now. And that’s saying something considering how much competition there is, with cryptocurrencies and NFTs leaping to mind as primary contenders.
The hype around AI has reached the point where it’s difficult to assess the truth or trustworthiness of many of the claims being made about it. Even the majority of industry execs are starting to take notice. In extreme cases, the hype can even warp into outright lies, as evidenced by Engineer.ai, an India-based software startup that was recently sued for falsely claiming to have created an AI app that was in fact driven by human labor instead of computers.
This hyperbole is nothing new. Since the dawn of computers, we have gleefully exaggerated and overestimated their abilities. As far back as 1965, AI pioneer and Nobel prize winner Herbert Simon said during an interview that “machines will be capable, within twenty years, of doing any work a man can do.” That was almost 60 years ago, and needless to say, Simon’s prediction has yet to come true.
Far from going the way of the ENIAC, this brand of overconfidence has continued to flourish. In 2008, futurist Ray Kurzweil stated that “by 2020, we’ll have computers that are powerful enough to simulate the human brain.” Computers have certainly come a long way since then, but surely not even the most ardent of AI enthusiasts would claim that they have reached the advanced state that Kurzweil prognosticated.
…or would they?
Artificial Intelligence: Where Are We and Where Are We Going?
There are those who believe that the Holy Grail of AI (a machine that can think, learn, and behave like a human) is not only inevitable, but will burst onto the scene any day now. There are even individuals who appear to believe that Strong AI is already here—albeit in an early stage of its development.
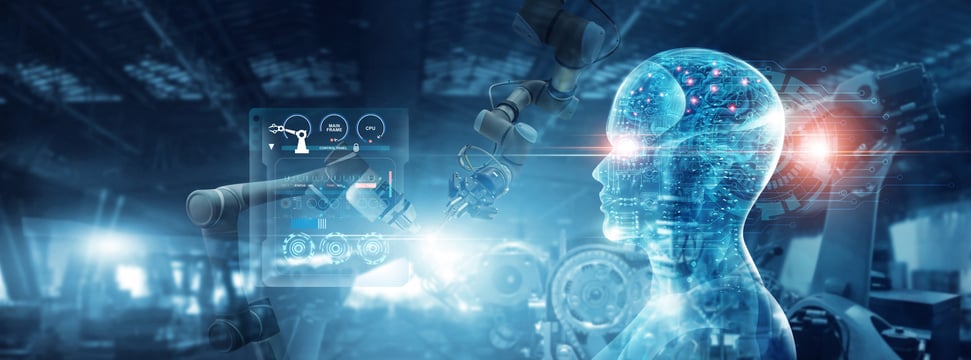
Dr. Nando de Freitas, a lead scientist for Google’s DeepMind AI project, recently took to Twitter to post a fiery retort to an opinion piece at The Next Web that expressed skepticism about the current vein of artificial intelligence research. As far as de Freitas is concerned, we’ve already reached the point where “The Game Is Over” in the search for an AI that can rival humans.
De Freitas’s confident proclamation comes on the heels of the recent unveiling of Gato, a DeepMind “generalist” AI system capable of executing an astonishing 600 different tasks. In the DeepMind scientist’s estimation, we will soon build a version of Strong AI (or AGI) simply by scaling up the systems that Gato and other deep-learning programs like OpenMind’s DALL-E 2 currently run on.
Of course, not everyone agrees with this assessment.
“Systems like DALL-E 2, GPT-3, Flamingo, and Gato appear to be mind-blowing,” wrote AI researcher Gary Marcus in response to de Freitas, “but nobody who has looked at them carefully would confuse them for human intelligence.”
Marcus went on to say that Gato and other deep learning programs, for all their successes, also regularly make errors that, if his children were to make, he would “no exaggeration, drop everything else I am doing, and bring them to the neurologist, immediately.”
So, who should we listen to? Where are we and where are we going on the path to AI? Is the game already “over”, as de Freitas claims? Or do we, as Marcus believes, still have a long way to go before humans–if ever–create a Strong Artificial Intelligence?
At the end of the day, there’s no way to know. Only time will tell which of these two outlooks is correct. The problem, however, is that this isn’t just a nitpicky online argument between academics about an abstract idea. How we think and talk about AI has enormous real-world consequences, and not just in the future but here in the present day. Globe-spanning and life-changing decisions are being made about this transformative technology right now, and we’re already seeing some of the negative impacts.
Artificial Bias
It’s no secret by now that human biases can, and often do, seep into the computer systems we create. Over the past several decades, the harmful effects of these biases have been most acutely felt by vulnerable and marginalized populations.
In recent years, facial recognition software has been exposed as racist. An Amazon recruiting algorithm was shown to favor men over women. Algorithms that decide who gets a mortgage and who doesn’t were revealed to be overwhelmingly discriminatory against people of color. In 2016, ProPublica investigated a criminal justice computer system in Florida that automatically mislabeled a majority of African-American defendants as “high risk” for committing future crimes, even when their offenses were minor in comparison to white defendants who were labeled “low risk.”
These are just a few examples of harmful bias in modern AI systems.
Cathy O’Neil is a computer scientist who, in her book Weapons of Math Destruction, criticizes our over-reliance on big data, warning of the dangers inherent in the view that automated, privately owned, “black box” computer systems can serve as objective and infallible arbiters of reality.
Here she is speaking during a TEDTalk in 2017:
…whereas an airplane that’s designed badly crashes to the Earth and everyone sees it, an algorithm designed badly can go on for a long time, silently wreaking havoc…
Algorithms don’t make things fair. They repeat our past practices, our patterns. They automate the status quo. That would be great if we had a perfect world, but we don’t...we have to consider the errors of every algorithm. How often are there errors and for whom does this model fail? What is the cost of that failure?
The Black Box Problem
Another awkward and troubling reality of modern artificial intelligence systems is that we often don’t really know how they work. This is what’s called the “black box” problem of AI, where the processes that govern our most advanced computer programs are so complex and opaque that they defy explanation. In some cases, not even the creators of certain machine and deep-learning systems can explain how their programs arrive at their conclusions.
This lack of transparency is unsettling for a number of reasons, particularly as we’ve handed more and more of society’s functions over to the inscrutable operations of machines. The opaquer a system, the more difficult it is to assess the intentions of its creators, and therefore whether or not the system is performing well or poorly in comparison to its programming, or whether it was designed in good faith to begin with.
By the same token, a lack of transparency makes it difficult to appeal the decisions of black box algorithms, leaving the human beings who are negatively affected with no recourse or justification for their predicament beyond a curt “Computer says no.”
Uncertainty: There Be Dragons Here
Finally, we come to the unknown region of the map where lie the not-yet fully realized existential threats that AI portends.
A common concern is automation. As our computers become more advanced, everyone from factory workers to doctors have grown increasingly fearful that their livelihoods are in danger, that there is a robotic workforce waiting in the wings to take their place. There is plenty of recent history to justify this concern, as many of the millions of people who lost their jobs during the pandemic did indeed end up replaced by automated machines. Meanwhile, the World Economic Forum estimates that as many as 85 million more people will be displaced by robots over the next five years. Tech and robotics companies have done little to assuage the anxiety surrounding this controversial issue, openly marketing AI as a viable alternative to virtually any job imaginable. We haven’t yet reached the threshold that Simon predicted, where computers are able to perform any task a human can, but AI researchers like de Freitas seem confident that that day is right around the corner.
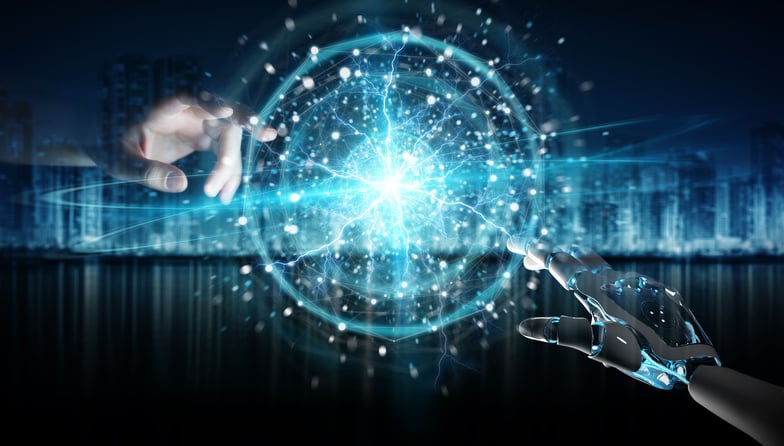
Then there’s the sci-fi stuff: The looming existential dread that a superintelligent AI might someday rise up and enslave humanity, or even try to wipe us out, Terminator style. These types of concerns, often voiced by the likes of celebrity personalities such as Elon Musk or Stephen Hawking, may warrant some skepticism of their own. But as we’ve already seen, computers need not have the intelligence of Skynet to still cause serious damage.
Indeed, the perils of artificial intelligence are not lost on AI researchers at the very cutting edge of their field. In their recent white paper, the team that developed DeepMind’s Gato flagged a number of harmful effects that advanced versions of modern AI could have if carelessly released into the wild. So-called “generalist” AI systems, they wrote, might be “exploitable by bad actors,” their powers harnessed for the express purpose of causing harm. Then there’s an echo of Oneil’s concern: that institutions and members of the public might end up vesting too much authority in thinking machines that, despite their impressive abilities, are still capable of deeply ingrained biases and grievous errors, “leading to misplaced trust in the case of a malfunctioning system.” The authors of the paper even appeared to lukewarmly suggest that modern AI systems might turn violent, resulting in “unexpected and undesired outcomes if certain behaviors (e.g. arcade game fighting) are transferred to the wrong context.”
In the end, the researchers concluded that given how little we still know about AI, there’s no way to provide a complete accounting of the risks involved, and that even a well-designed AI could result in negative impacts beyond anyone’s ability to foresee.
Bottom Line
There’s no question that computers have soared to extraordinary heights. Machine and deep-learning processes can now write poetry, pilot construction vehicles, and perform an ever-expanding array of dizzying computational and analytical tasks. Given the rapidly accelerating state of digital technology, the meteoric ascendance of artificial intelligence–whichever version of it you subscribe to–can seem inevitable at times.
A grounded perspective, however, recognizes that AI still has a lot of bugs to work out. Ambiguous terminology can sow confusion and hyperbolic sales pitches can lead to overblown promises at best, or deliberate distortions of the truth at worst. Meanwhile, failure to reconcile where AI research is and where it’s going has serious implications for how this technology is used now and into the future. Biased “black box” algorithms are an example of how current versions of AI are already resulting in widespread harm, and there are many more potential dangers waiting for us on the horizon.
None of this is to say that AI ought to be abandoned or slowly lowered into a vat of molten steel. Academics, scientists, and researchers in the AI field can combat confusion and rein in hype by being clear and consistent in their communication about what exactly AI is and is not. Consumers, industry leaders, and members of the public should also seek to educate themselves about a technology that could already be having an outsized impact on their lives and livelihoods. Creators of AI must meanwhile do their utmost to infuse their systems with ethics, eliminate bias, and build transparency into their machines. Moving forward, computer scientists and industry leaders ought also to ask themselves whether or not AI should be the go-to solution for every problem under the sun. Machine-learning algorithms are great at preventing kickback in power tools, but they probably shouldn’t be put in charge of predicting future criminals or deciding who gets a home and who doesn’t.
The future of AI is uncertain. Wherever this path takes us, we can ensure safer and more equitable outcomes by treading carefully, thinking critically, and tempering our excitement with clear-eyed curiosity about what kind of world we want our machines to help us build.